We are pleased to inform you that the recent work "General Board Game Playing for Education and Research in Generic AI Game Learning" of Prof. Wolfgang Konen has been accepted for publication and it will be presented as a Poster at IEEE CONFERENCE ON GAMES (CoG) 2019. CoG 2019, held in London, UK, will be hosting many peer-reviewed paper and poster presentations as well as academical and industrial invited talks in various game technology related fields. Wolfgang Konen introduces a general board game playing and learning framework, in his recent paper. You can find more information about the developed educational tool by Wolfgang Konen in one of our former blog-posts.
Recommender System is the name given to any software designed to recommend you objects to purchase, to click on or to watch, which might be attractive to you with a high probability. Development of such systems became a hot topic for software developers and machine learning engineers in the last years. A well-performing recommender system can make significant contributions to various online platforms.
Nowadays, many of us interact with recommender systems on a daily basis. If you have an account in Amazon or Netflix or many similar online services, you may have received suggestions that often fit your interest. This can vary from a movie suggested by Netflix or very various products suggested by Amazon. The targeted advertisements on Facebook or the targeted pop-up advertisements in your browser are also other examples of applications of these systems. Almost no two person receives the exact same advertisements or suggestions on an online platform. All these personalized suggestions and advertistments use recommender systems to find the best match for your interests according to your former ratings, purchases or clicks.
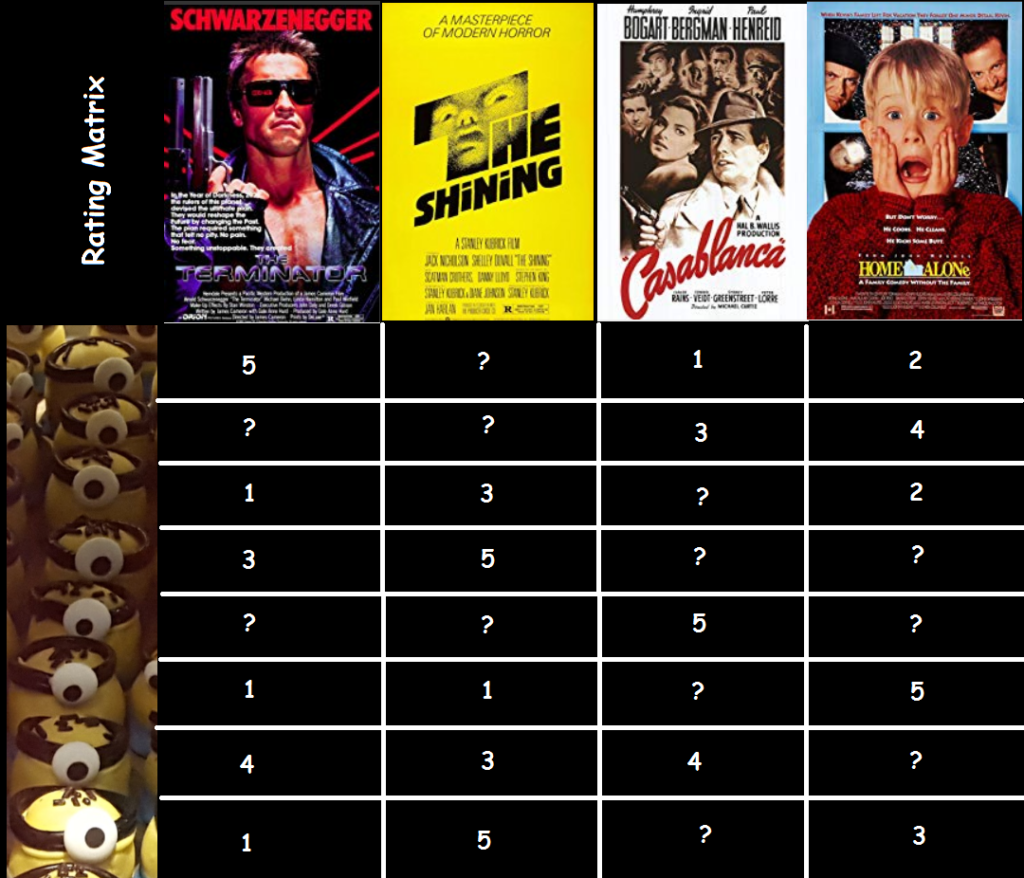
Content-based recommender systems often make use of the explicit features of the users and the items to provide recommendations. In contrary, the Collaborative Filtering recommender systems do not have access to any sort of explicit features and tend to learn the important features of users and items implicitly. The real-world recommender systems are often a hybrid of both approaches.
You can try our movie recommender system demo, by simply giving your own ratings and see what the system will suggest to you. This movie recommender system is based on the implementation of Matrix Factorization algorithm in Python. Matrix Factorization is a collaborative filtering approach which tends to learn implicit features for users and items by factorizing the exiting rating matrix. We expalin this algorithm in more details, in this Google Colaboratory Notebook . We describe how the recommender system problem turns into an optimization problem and how to handle such optimization tasks. Also a step-by-step implementation of this algorithm in Python is provided.
On Saturday, May, 25th, 2019, TH Köln hosted the annual Digital Xchange conference, which is organized as a cooperative work of Opitz Consulting and TH Köln, for the third time. This 1-day conference is becoming more and more popular: this year it attracted more than 800 participants. More than 100 talks were given in the field of digitalization and its impact on industry. The topics had a large variety and covered many areas of digitalization including Big Data, Cloud Computing, Industry 4.0, Machine Learning, Artifical Intelligence, etc. You can find a list of all talks here.
One of the very interesting talks at Digital Xchange which attracted a large group of audience was "Deep Learning mit Keras und TensorFlow" given by Henning Buhl. He is a Computer Science Bachelor student at TH Köln who also works part-time under supervision of Prof. Wolfgang Konen. His talk coverd a large range of themes from neural networks to machine learning and Python code examples. As part of his talk he gave a live demo on Google Colaboratory with Jupyter notebooks showing TensorFlow and Keras examples. These examples are available on GitHub for your own study if you like. You can find them here or here on GitHub.
This year the 28th Computational Intelligence workshop took place on November, 29th-30th, in Dortmund. As every year, this two-day workshop was packed with many interesting talks and two gripping keynotes (deep learning and OpenML). The presentations and the keynotes had diverse topics including fuzzy control, surrogate-assisted optimization, modeling techniques, deep learning, interesting applications (e.g. gait recognition, driving lane recognition) and several other areas
The deep learning and reinforcement learning summer schools hosted by Canadian Institute For Advanced Research (CIFAR) and the Vector Institute are among the most prestigious summer schools in the field of machine learning and artificial intelligence. This year the summer school was taking place in Toronto, Canada from 25th of July till 3rd of August including 7 days of deep learning school and 3 days of reinforcement learning lectures. Since deep learning became very popular in the last years, a large number of students and researchers (over 1200 applicants) applied to participate in both of these summer schools and a little bit more than 250 delegated to attend. This year two of the PhD students from TH Köln were among the 23% lucky attendees of these valuable summer schools.
Every day of the summer schools was planned with many interesting lectures, contributed talks and a poster session. The lectures covered a wide variety of topics. Also the level of the lectures were varying from basics going to very advanced topics. The lecturers were from DeepMind, Google Brain, Microsoft Research, Vector Institute as well as pioneers of the field from University of Toronto, University of Alberta and the Montreal Institute of Learning Algorithm (MILA) and several other active institutes in the field. The contributed talks were given by PhD students and were selected by the summer school's organizers committee. Samineh Bagheri and Markus Thill from TH Köln also had a chance to present a poster in this summer school.
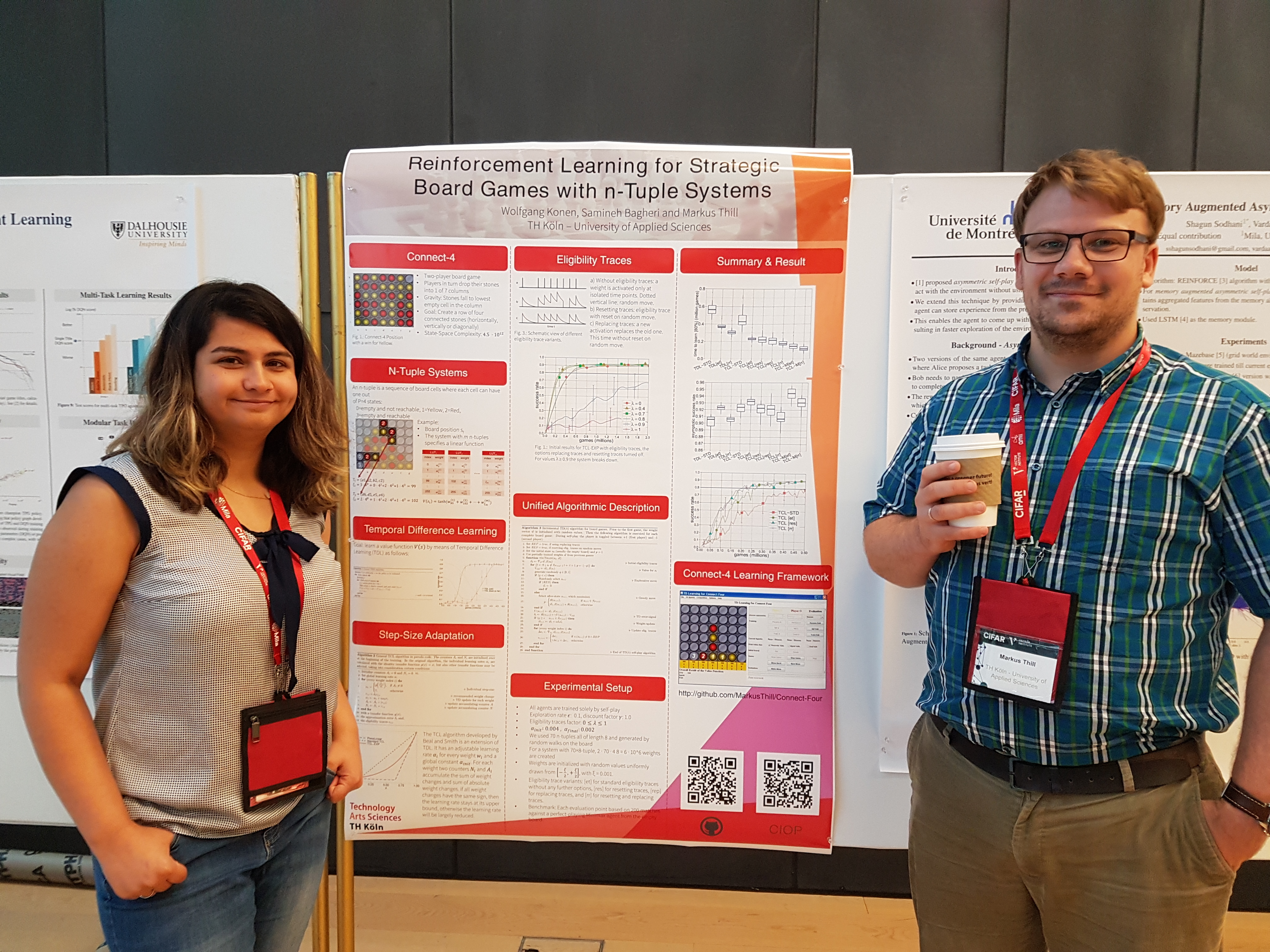
We are grateful for partial funding of travel and conference costs by the deanery of the faculty and the computer science institute.
ECDA is an international conference with the main focus on data science and data mining which is being held in different European cities since 2013 almost every year. This year the conference was held in Paderborn, Germany. This 3-days conference hosted many interesting keynotes and a large number of talks given by scientists from all over the world. Prof. Wolfgang Konen organized and chaired the "Time Series Analysis and Online Algorithms" session. In the same session Markus Thill presented his recent work "Online Adaptable Time Series Anomaly Detection with Discrete Wavelet Transforms and Multivariate Gaussian Distributions" and he received valuable remarks and feedback. The deadline for the paper submission is on 15th of September and after successfully passing through a reviewing process the work will be published in Archives of Data Science, Series A Journal.
Digital Xchange Conference was held on 23rd of July in TH Köln, Campus Gummersbach as a collaborative work of Opitz consulting company and TH Köln. The event attracted over 600 audience and the talks covered a large area of digitalization field including cloud computing, artifical intelligence, industry 4.0, augemented reality, IT security and many more interesting topics.
"Machine Learning and AI for Predictive Maintenance" is the title of an interesting presentation given by our research team member Mr. Markus Thill. Markus Thill presented an overview of his PhD research topic which he is pursuing at TH Köln under the supervision of Prof. Wolfgang Konen. He discussed several anomaly detection algorithms and their performances. The talk attracted a lot of attention from the audience.
The R package TDMR (Tuned Data Mining in R) is now available on CRAN in a major new release 2.0. It supports the new R package SPOT 2.0 (Sequential Parameter Optimization Toolbox) with its largely redesigned and simplified interface. TDMR 2.0 has as well a simplified interface. TDMR documentation and TDMR tutorials have been rewritten to account for the simpler interface.
Tuned Data Mining in R ('TDMR') performs the complete tuning of a data mining task (predictive analytics, that is classification and regression). Preprocessing parameters and modeling parameters can be tuned simultaneously. It incorporates a variety of tuners (among them 'SPOT' and CMA with package 'rCMA') and allows integration of additional tuners. Noise handling in the data mining optimization process is supported, see Koch et al. (2015) <doi:10.1016/j.asoc.2015.01.005>.
More information on TDMR is available on the TDMR project page.
GBG (General Board Game Playing & Learning) is an Open Source software framework developed at TH Köln, University of Applied Sciences. GBG aims to ease the entry for the students into game learning and the reinforcement learning research area which is a very interesting sub-field of artifical intelligence. In 2018,